Prashant Sardeshmukh
CIRES Senior Research Scientist
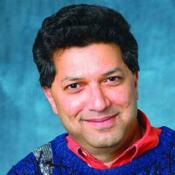
- Ph.D., Princeton University, 1982
Research Interests
I am a climate dynamicist with broad interests in the diagnosis, modeling, prediction, and potential predictability of large-scale weather and climate variations around the globe on time scales of days to centuries. Our team is engaged in documenting and understanding the regional aspects of ongoing climate changes, especially changes in the statistics of extreme weather, and determining to what extent they are anthropogenic or consistent with natural climate variability. We are also interested in documenting and understanding the actual and potential skill of weather and climate predictions, which is inherently limited due to the chaotic nature of the system, and how current prediction systems need to be improved to achieve the potential skill.
Current Research
Sensitivities of the global weather forecasting system used at the National Centers of Environmental Prediction (NCEP)
The large improvement in weather prediction skill over the past several decades has been described as a “quiet revolution” resulting from many small steps rather than a few dramatic leaps. One has now apparently entered a stage of diminishing returns in skill improvement, with no clear guidance as to improving which aspects of current forecast systems will yield the greatest benefit. Broadly speaking, forecast systems have three basic elements: 1) the input observations, 2) the data assimilation (DA) method used to merge those observations with model-generated guess fields to create the forecast initial conditions, and 3) the forecast model itself. As forecast systems continue to evolve, it will remain important to assess their relative sensitivities to these three elements to help set priorities in system development. In a recently published study (Wang et al, Monthly Weather Review, 2019) we investigated these sensitivities of the current Global Forecast System (GFS) of the National Centers for Environmental Prediction (NCEP). Four parallel sets of 7-day ensemble forecasts were generated for 100 forecast cases in mid-January to mid-March 2016. The sets differed in their 1) inclusion or exclusion of additional observations collected over the eastern Pacific during NOAA’s El Niño Rapid Response (ENRR) field campaign, 2) use of a “Hybrid 4D-EnVar” versus a “pure EnKF” DA method to prepare the initial conditions, and 3) inclusion or exclusion of “stochastic parameterizations” of chaotic physical processes in the forecast model. In general, the forecast errors were found to be only slightly sensitive to the additional observations, more sensitive to the DA method, and most sensitive to the inclusion of stochastic parameterizations in the model, which reduced errors globally in almost all the variables considered. The reduction in the precipitation forecast errors were particularly striking.
Research Categories
Atmosphere, Climate and Weather, OceansHonors and Awards
to
Invalid date -Sponsors
-
Invalid dateP.I.(s)
About CECA
CECA connects and creates a supportive environment for graduate students and postdocs who come from various academic units to do research in CIRES.