Eric James
Gov Rep
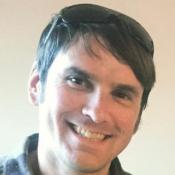
- Master of Science, Atmospheric Sciences, Colorado State University, 2009
- Bachelor of Science, Geography, University of Utah, 2006
- Bachelor of Science, Meteorology, University of Utah, 2006
Research Interests
I joined CIRES in 2010 as a research associate within the NOAA Global Systems Laboratory’s Assimilation and Verification Innovation Division (formerly the Assimilation Development Branch). Our group is located in the David Skaggs Research Center at the Department of Commerce Labs in Boulder, Colorado.
The primary goal of our group at NOAA, along with our sister division the Earth Prediction Advancement Division, is to advance the state of the science for short-range, regional numerical weather prediction (NWP). We seek to find the best ways to simulate atmospheric phenomena through both explicit representation and parameterization of processes, as well as the optimal way to initialize numerical forecasts. We collaborate with NOAA’s National Centers for Environmental Prediction (NCEP) to transition our experimental forecast systems into an operational environment. Over the previous 10 years, the focus of our group has been on the development of the mesoscale Rapid Refresh (RAP; 13km horizontal grid spacing; https://rapidrefresh.noaa.gov/RAP/) and convection-allowing High-Resolution Rapid Refresh (HRRR; 3km horizontal grid spacing; https://rapidrefresh.noaa.gov/hrrr/HRRR/) systems, which use the community-supported Weather Research and Forecasting – Advanced Research WRF (WRF-ARW) and Gridpoint Statistical Interpolation (GSI) software packages. The RAP and HRRR systems are used daily by operational and private weather forecasters to anticipate high-impact weather events for a variety of weather-sensitive sectors, including aviation, renewable energy, firefighting efforts, hydrologists, and emergency managers. Efforts are now transitioning to development with NOAA's new dynamical model core, the Finite Volume Cubed (FV3) for a Rapid Refresh Forecast System (RRFS) to replace the HRRR in the 2023-2024 timeframe.
My research interests within the Assimilation and Verification Innovation Division have been focused mainly on quantifying the forecast skill impacts of various types of weather observations. My work on this topic has involved extensive sets of data denial experiments, more formally referred to as observation system experiments (OSEs), wherein individual observation types are systematically denied from the forecast and assimilation system for a period of time considered sufficient for statistically-significant results (generally 1-2 weeks per season). An article describing a set of OSEs using the third version of the RAP system (RAPv3) was published in Monthly Weather Review in 2017. This work also led to insights regarding the configuration of data assimilation for surface observations, as well as specific parameters within the non-variational cloud analysis used in the RAP and HRRR systems. A significant outcome of this work was the result that weather observations from commercial aircraft (via regional AMDAR systems) are the most valuable source of upper-air observations for the RAP NWP system. We are also working to document, in a controlled experiment, the impact of reduced aircraft traffic during the COVID-19 pandemic upon regional forecast skill, which has been a topic of great interest over the past year. I am also interested in upcoming new sources of aircraft observations, including Mode-S and EHS, which may provide major benefits for NWP systems if we are able to assimilate them.
The availability of an adjoint model, or, alternatively, an ensemble data assimilation system, permits the development of a different type of observation sensitivity framework: the forecast sensitivity – observation impact (FSOI) framework. Such systems, routinely used by many worldwide national centers which run global NWP models, allow for quantification and attribution of model forecast error reductions due to different observation types. The FSOI framework is computationally inexpensive and can be run routinely, but has not been widely applied for regional NWP systems. Our group is investigating the feasibility of setting up an FSOI framework for the RAP/HRRR system, or its operational successor.
A secondary major research interest of mine is in quantitative precipitation forecasting (QPF), particularly for intense rainfall events and flash floods. In 2016-18, our group collaborated with the Physical Sciences Laboratory in our lab, as well as two private Colorado companies, in the Colorado – New Mexico Regional Extreme Precipitation Study. The goal of the study was to revise dam engineering standards throughout the states of Colorado and New Mexico, including dams in high-elevation watersheds, based on the maximum probable amount of precipitation for various time durations. The project led to a detailed examination of a five-year archive of HRRR forecasts, covering the entire continental United States, and its utility for supplementing the limited rain gauge and radar-derived quantitative precipitation estimates (QPE) in the complex terrain of the western US. The lack of ground truth rainfall data was a recurring theme in the study, and much additional work is needed to determine precipitation climatologies in the western US. Our group received an award from the Colorado Governor for this research.
Stemming from this research, I am also interested in quantifying uncertainties in QPE in regions of sparse observational coverage. As has been pointing out in numerous studies, high-resolution QPF may provide more valuable information on precipitation in regions of complex terrain than do the available observations. A data assimilation framework provides an elegant and attractive path towards combining QPF and QPE in an optimal way to account for uncertainties inherent in both sources of information, and I am working towards a preliminary capability to represent this.
My third major area of work in the past few years has been in the development and support of the real-time HRRR-smoke system. The HRRR-smoke system is based on the meteorological HRRR NWP system, but it forecasts wildfire smoke plume behavior based on polar-orbiting satellite fire detections, as well as emission and plume rise parameterization. The real-time HRRR-smoke has proven to be an invaluable tool for air quality forecasters and emergency managers over the past several fire seasons, and I have played a key role in maintaining and developing the real-time system. The greatly increased visibility and pressure on the experimental HRRR-smoke system over the summer of 2018 made it clear that the tool needs to be transitioned into operations; the smoke forecasting capability is slated for inclusion with the final implementation of the meteorological RAPv5 and HRRRv4 NWP systems. The RAP-smoke (https://rapidrefresh.noaa.gov/RAPsmoke/) and HRRR-smoke (https://rapidrefresh.noaa.gov/hrrr/HRRRsmoke/) have also emerged as important research tools, and we have extensive collaborations with other atmospheric chemistry and air quality research groups around the country. Work is ongoing to also develop a corresponding volcanic ash forecasting capability for the North American domain RAP system, as well as developing a similar capability for forecasting blowing dust. Visibility reductions from smoke and blowing dust pose a major transportation challenge in the western US, particularly in recent years with the catastrophic and frequent wildfire activity.
Research Categories
Atmosphere, Climate and Weather, Energyto
Invalid date -Sponsors
-
Invalid dateP.I.(s)
About CECA
CECA connects and creates a supportive environment for graduate students and postdocs who come from various academic units to do research in CIRES.